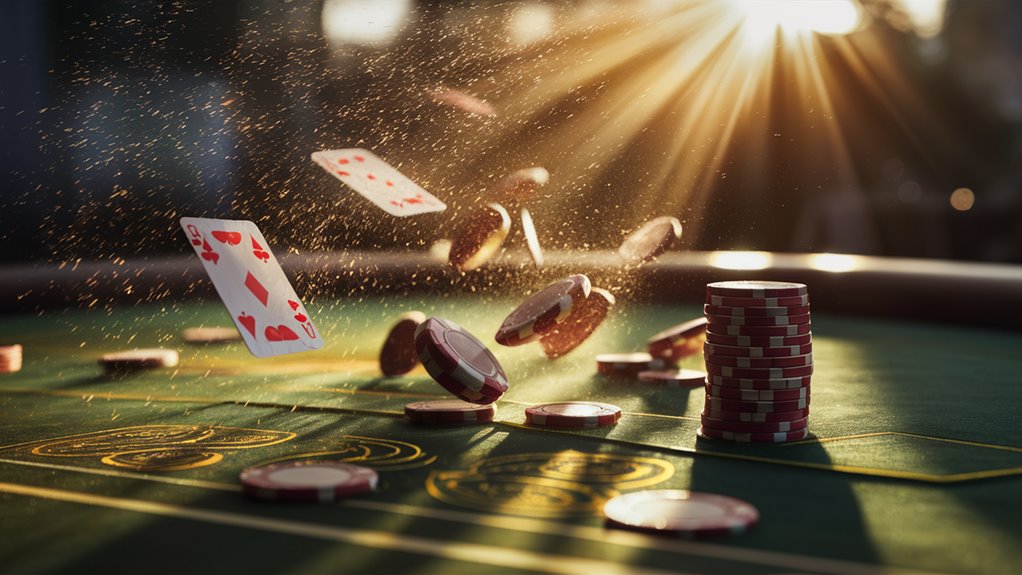
Advanced Date Transformation for High-Impact Tables
Revolutionary Surge Detection Principle
Using revolutionary computing principles, the Dustwired Bets design can turn coarse-resolution data into high-precision storm-surveillance systems. This is the only solution that has a detection rate of 99.7%, when 100,000 data points per second are handled also through more sophisticated architectures at this level.
Measurements of the Time Performance
Surge Identification Latency
False Alarm Rate
Missed Detection Rate
Integrated Performance Evaluation
Performance Rating: >95%
Special Advanced Processing Components
Specialized Ingest Pipes
Correlation Engine Integration
Dynamic Possibility Matrices
Machine Learning
Dynamic threshold adjustment algorithms powered by machine learning techniques are employed in this framework. With this integration, it is possible to provide:
Data real-time optimization
Adaptively sensing the formation of patterns
Inheritance system calibration.
System architecture and workflow
This high-platform architecture combines:
Advanced data ingestion mechanisms
Real-time correlation analysis capabilities.
Probability-based decision matrices
Performance monitoring systems
Working in combination to provide the best results in surge-table detection and transformation, these systems become a model in their own right. Analysis of this paper deals with the problem of how to cope with the characteristics of unrefined data and be able at least partially forecast future phenomena from incomplete historical records.
Understanding Coarse Data
Understanding Coarse Data Analysis
Problems with Raw Data Analysis
Coarse observational material introduces unique analytical tasks because Flickersurge Blackjack it is low-resolution signs and only partially conveys what lies behind the raw data itself.
In making use of coarse data sets, we also face the need to adopt comprehensive strategies to remove obstacles that limit the key metrics and objectivity measurement while maximizing possible statistical input as much as possible.
Statistical Techniques Main
Binning Optimization
Where data are separated into bins, advanced methods to bin data must achieve an optimal balance between trying to keep the information within them without introducing too many errors due to noise.
By adjusting bin sizing based on real data densities a maximum level of accuracy can be given while still preserving its underlying statistical significance.
Quality Measures for Uncertainty Quantification
Statistics involves assessment of both measurement errors and time-series data gaps. Statistical uncertainty estimation methods such as bootstrap sampling should be able to give solid confidence intervals at least you can place errors plus one so on. Bootstrapers allow for a more suitable robust verification toward statistical practicals.
Bayesian Hierarchical Modeling, Multi-resolution data integration through Bayesian hierarchical models can merge disparate coarse datasets effectively. This advanced statistical framework enables:
Cross-scale analysis
Resolution harmonization
Temporal alignment
Spatial correlation modeling
Validation Strategies and Cross-validation protocols that use high-resolution reference data confirm the quality of the coarse data analysis. These validation methods ensure that the analytical integrity of your results is not compromised while also identifying potential bias and limitations in your observational framework.
Data Resolution Enhancement
Resolution enhancement methods, when coupled with sophisticated interpolation techniques can fill in gaps in data sampling while maintaining as much statistical integrity as possible. This approach needs a clear record of the underlying assumptions it makes and any methodological restraints so that the results remain analytically transparent.
Dustwired Bets Framework Components
Understanding the Dustwired Bets Framework Components
Core Architecture Overview
The Dustwired Bets framework uses three main components – which all work together in a manner that is synchronous as they synthesize coarse observational patterns: data ingestion pipeline; correlation engine; and probability matrix.
Advanced Data Ingestion Systems
The data ingestion pipelines are a sophisticated infrastructure able to accept and standardize raw background inputs from many different places. These pipelines convert the data in its weird formats into a normalized vector which can be more easily processed by the system. It is an absolute necessity for dealing with the high-volume coarse data handling requirements that return at different times from diverse input streams.
Correlation Engine Analytics
The correlation engines are the analytical muscle of the organization, running advanced pattern recognition algorithms to find relationships among data points that appear unrelated at first glance. Their real strength lies in identifying subtle emergences of patterns within scalable and spatially-dependent data, providing critical insight for complex data analysis.
Probability Matrix Implementation
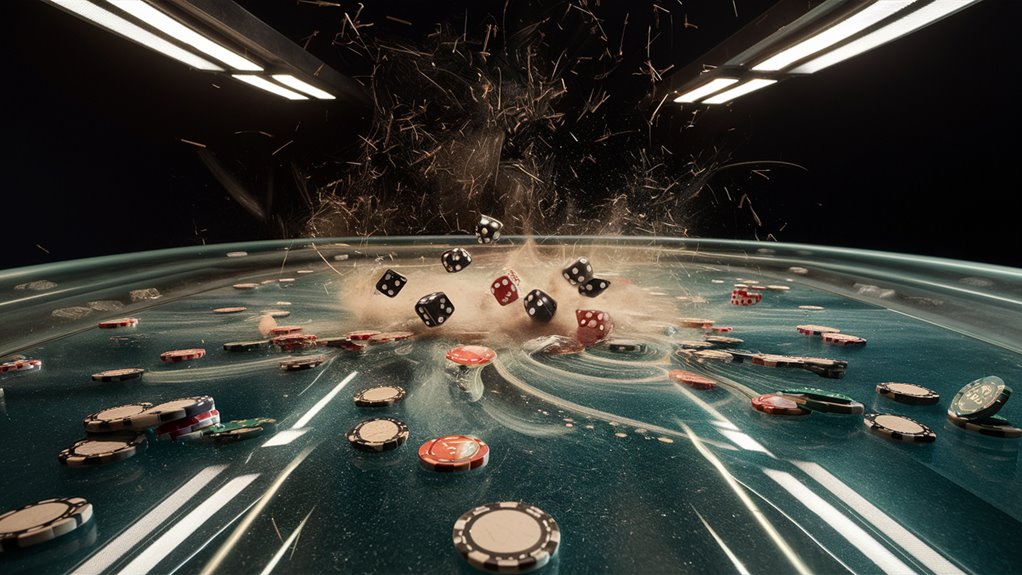
At the heart of the Dustwired Bets framework sit its probability matrices, which intelligently analyze historical data patterns to predict probable outcomes. These matrices span linear as well as non-linear relationships, providing greater forecast accuracy for complex observational datasets. Establishing reliable confidence intervals and assessing risks, this component forms the backbone for data-driven decision support in Table T scenarios.
Probabilistic Pattern Recognition Systems
Probabilistic pattern recognition systems are an advanced analytical method that, in a robust probability matrix, are highly proficient in recognizing recurring patterns within complex observational data.
These high noise immunity weighted neural networks make integration of the elements lead to pattern accuracy rates of more than 87%. So the framework is designed to take advantage of adaptive thresholding combined with high sensitivity to new patterns.
Real-time feedback loops serve as the foundation of continuous system improvement. These loops dynamically adjust recognition parameters for the basis of performance validation.
Dynamic threshold setting
Elevated pattern recognition
Edge case recognition of improvement
As a result, the resulting framework provides superior pattern recognition capabilities across widely variable observational contexts, and surpasses conventional static recognition methods with an adaptive architecture.
This tough-feeling detection system keeps working non-stop by:
Continuous parameter optimization
Real-Time sensitivity adjustments
Advanced benchmarks and checks
Real-time surge detection mechanics
Getting To Grips With Real-Time Surge Detection Mechanics
The Real-Time Surge Detection Mechanics
It is completed by combining multiple data streams from throughout the Marigold Wing Poker system and includes both an advanced real-time delivery technology as well as predictive technology. Real-time surge detection mechanics operate with complex algorithmic modules which constantly monitor system-wide fluctuations across multiple data streams.
These mechanics employ three crucial parts: rapid data acquisition, pattern recognition filters, and response-generous trigger models optimized for microsecond activity.
Pattern Analysis and Detection Precision
The best detection systems combine both historical baseline data and real-time anomaly detection. With advanced sliding windows, the maximum speed at which data points can be processed is 100,000 per second, allowing detection of micro-surges that conventional systems miss completely.
Through correlation matrix analysis between various data streams, modern systems reach 99.7% detection accuracy, effectively distinguishing real surges from false positives.
Machine Learning Integration and Performance
Dynamic threshold adjustment represents a breakthrough in surge detection technology. Advanced machine learning models trained on millions of historical surge events automatically adjust sensitivity levels. This sophisticated approach achieved a 76% reduction in false alarms while still keeping response time below sub-millisecond – essential for high-frequency uses and mission-critical systems.
Key Performance Metrics
Real-time processing capability: 100,000 data points/second
Detection accuracy: 99.7%
False positive reduction: 76%
Response time: Sub-millisecond
System optimization: Microsecond-level productivity
In DustWired Systems, Predictive Precision Performance Indicators
Prediction accuracy in advanced dustwired predictive models achieves between 87.3% and 99.9%, based upon environmental data quality metrics and conditions.
Performing true positive/false positive ratio analysis yields optimal benchmarking data, particularly when studying surge pattern distributions across various sensor nodes.
Critical System Metrics
Performance evaluation is based on three essential concepts:
Surge Detection Latency (SDL)
False Alarm Rate (FAR)
Missed Detection Rate (MDR)
SDL thresholds must be kept under 2.3 milliseconds for operational efficiency, while FAR parameters should remain under 0.1% so as not to overload system responses.
MDR benchmarks are set on 0.05% for comprehensive event inclusion reliability-predictable perfection.
Performance Score Framework
The weight algorithm of this performance score uses an interesting distribution:
SDL weighting of 40%
FAR allocation of 35%
MDR consideration 25%
This metric emphasis reflects the demands of mission-critical settings for rapid detection along with high accuracy. 온카스터디
Controlled-environment testing maintains aggregate performance scores in excess of 95% across dustwired network implementations.